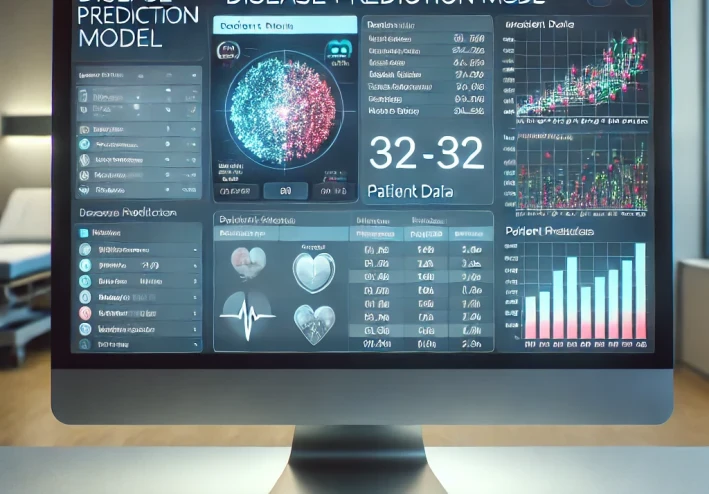
Deep Learning in Medical Imaging
Deep Learning involves the use of neural networks with multiple layers to learn from vast amounts of data. Unlike traditional machine learning, which often relies on manual feature extraction, DL can automatically identify features from raw data, making it particularly effective for image analysis. This ability to process and interpret images accurately and efficiently is transforming medical diagnostics.
Common DL algorithms used in medical imaging include Convolutional Neural Networks (CNNs), which specialize in image processing. CNNs are adept at identifying patterns and abnormalities in medical images, such as tumors or fractures. Recurrent Neural Networks (RNNs), on the other hand, are used for analyzing sequential data, such as ECG or EEG signals, while Generative Adversarial Networks (GANs) are employed for image enhancement and generation.
The applications of DL in medical imaging are numerous. In radiology, DL algorithms analyze X-rays, CT scans, and MRIs to detect conditions like pneumonia, tumors, and brain abnormalities. These systems assist radiologists by providing second opinions and highlighting areas of concern, which can lead to early detection and improved outcomes. In pathology, DL is used to analyze slides, aiding in the diagnosis of diseases such as cancer. Ophthalmology also benefits from DL, with systems capable of detecting eye diseases like diabetic retinopathy from retinal images.
Successful implementations of DL in medical imaging are evident in various case studies. Google DeepMind Health, for instance, uses DL to diagnose eye conditions with remarkable accuracy at Moorfields Eye Hospital. Enlitic, a startup specializing in medical imaging, employs DL to analyze images and has demonstrated significant improvements in diagnostic accuracy.
DL offers several benefits in medical imaging. It improves accuracy by detecting subtle patterns in medical images that may be missed by human eyes. This leads to earlier and more accurate diagnoses. DL also enhances efficiency, processing large volumes of images quickly and reducing the workload on healthcare professionals. Moreover, DL systems provide consistent results, minimizing variability in diagnosis.
Despite its potential, DL in medical imaging faces several challenges. One major challenge is the significant computational resources required to train and deploy DL models. Additionally, the quality and diversity of training data are critical for model accuracy. Ensuring that DL models are trained on representative datasets is essential to avoid biases and inaccuracies. Ethical and practical issues, such as patient privacy and data security, also need to be addressed.
Looking to the future, the prospects for DL in medical imaging are bright. Ongoing research aims to enhance algorithm accuracy, integrate DL with other technologies, and make DL systems more accessible. Innovations such as explainable AI (XAI) are being developed to make DL decision-making processes more transparent to clinicians. As these technologies continue to advance, their impact on patient care is expected to grow significantly.
In conclusion, Deep Learning is transforming medical imaging by providing accurate, efficient, and reliable diagnostic tools. The integration of DL in healthcare can lead to significant improvements in patient outcomes and reduce the workload on healthcare professionals. Embracing DL in medical imaging is a step towards more effective and personalized healthcare.
